Twelve industry leaders across the automotive and automated driving technology spectrum, including carmakers Audi, BMW, Mercedes, Volkswagen and Fiat Chrysler Automobiles, and technology firms Aptiv, Baidu, Continental, HERE, Infineon and Intel, have developed an industry-wide definition of safety with the July 2, 2019, publication of a white paper entitled “Safety First for Automated Driving” (SaFAD).
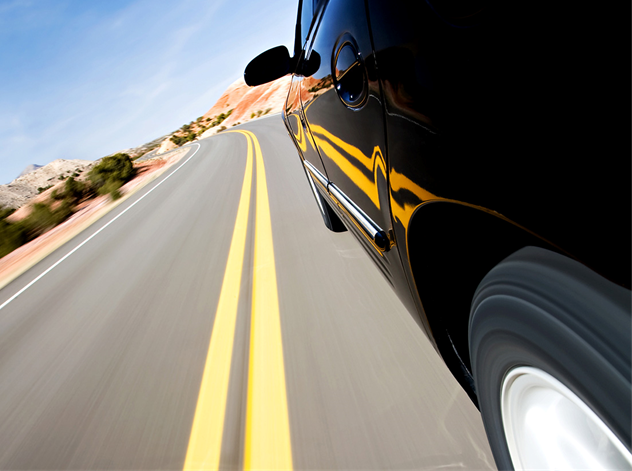
The publication addresses relevant safety topics for automated driving, from safety by design to the verification and validation processes in the context of Level 3 and Level 4 automated driving. In short, its aim is to highlight the safety- and security-relevant aspects of developing, producing, operating and maintaining self-driving vehicles, with the intention of working towards a standardization of automated driving, building on the work that was already done for Level 1 and Level 2 driver assistance systems.
People often ask when will automated driving come to the market and will it look like. The upcoming new features of automated mobility are the (1) Level 3 traffic jam pilot, (2) Level 3 highway pilot, (3) Level 4 urban pilot and (4) Level 4 car park pilot.
The traffic jam pilot is already available in in a Level 2 version in many recent car models, meaning it always requires the awareness and supervision of the driver. When it is Level 3, given a specific ODD (operational design domain), the driver will be able to divert his or her attention away from the traffic and concentrate on the entertainment system or read materials for the office.
The Level 3 highway pilot will allow the driver to log in on traffic on a highway and hand over control to the Level 3 driving system within the ODD “highway,” and the driving system will alert the driver if necessary and hand back control if needed. The driver needs to be present and prepared to overtake control when required.
The Level 4 parking pilot will come to the market shortly, given that the lower speed of the car control on the moving car can be realized and safeguarded relatively easy.
The Level 4 urban pilot is more complicated to manage as it is intended to work in cities. What’s more, as the “Level 4” indicates, the urban pilot is, given a specific ODD, is “fully automated” driving (i.e., a human driver can still request control, and the car still has a cockpit), rather than “full automation,” (i.e., the driver doesn’t need to be fit to drive and don’t even need to have a license; the car performs any and all driving tasks and there isn’t even a cockpit).
Achieving a uniform degree of safety
It is currently impossible to achieve a necessary and uniform degree of safety because each country has its own regulatory standards (and different regulatory standards may exist within a country) and also because there are no development standards for automated driving. In response, SaFAD advocates for a “safety by design” method, which approaches automated driving from an analytical perspective, addressing system safety by designing for scenario-based behaviors, and addressing technological capability by analyzing use cases and scenarios to design a robust and safe system. In difficult environments the car should always act with minimal risk maneuvers and under minimal risk conditions, the purpose being to bring the vehicle to a tolerable risk level.
Road safety is the ultimate goal
The purpose of self-driving vehicles is not innovation for innovation’s sake. Rather, the aim is to have a positive effect on safety on public roads. According to research, most accidents are caused by human error, and there is an urgent need to reduce the rate of fatal accidents. Automated driving can help to, if not eliminate, at least reduce the risk of crashes.
What is SOTIF?
SOTIF is the shorthand for the new ISO/PAS 21448 standard. ISO/PAS 21448 applies to functionality that requires proper situational awareness in order to be safe, and the standard is concerned with guaranteeing “safety of the intended functionality,” or SOTIF, in the absence of a fault. (This is in contrast with traditional functional safety, which is concerned with mitigating risk due to system failure.) SOTIF provides guidance on design, verification and validation measures (i.e., systematic identification, evaluation and risk mitigation.) It maximizes known safe behavior by identifying potential risks via analysis. It minimizes the known potential unintended scenarios by simulating function and identifying where improvements can be made. And it minimizes unknown unintended scenarios through endurance and driving tests.
Cyber security and safety overlap
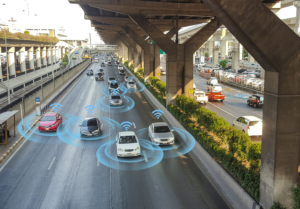
Because security focuses on the system’s ability to resist some forms of unintentionally malicious action, the analysis tools and mechanisms (e.g., data integrity) also affect safety. Cyber security in the context of automated driving is so important because cars connect with other cars or its environment.
Baseline requirements
According to SaFAD, any automated driving system must have the following basic set of system capabilities:
- Determine location: The system should be able to determine location, whether inside or outside, relative to the operational design domains (ODDs), using sensor information and external (map) information (including vehicle position on this map and on the street), to facilitate localization.
- Perceive relevant static and dynamic objects: All entities that an automated driving system needs to be aware of for its functional behavior should be perceived, optionally pre-processed and provided correctly, including, most importantly, static instances (e.g., road boundaries), dynamic objects (e.g., other cars or vulnerable road users) and other obstacles exceeding critical size.
- Predict the future behavior of relevant objects: The relevant model should aim to create a forecast of environment by interpreting relevant objects to predict future motion and the overall scene.
- Create a collision-free and lawful driving plan: From a lawyer´s perspective, probably the most challenging part is to educate the car to behave like a reasonable human being.
- Maintain a safe lateral and longitudinal distance to other objects (after accurate sensoring and localization, considering its own motion properly);
- Comply with all applicable traffic rules within the ODD: machine-interpretable traffic rules need to be programmed into the system, allowing the vehicle to obey the rules in order to produce a lawful driving plan (there is a challenge in this, as rules vary from jurisdiction to jurisdiction, from state to state, and even from municipality to municipality);
- Consider potential areas where objects may be occluded (i.e., consider unforeseeable risk);
- In unclear situations the right of way is given, not taken (safety first!);
- If a crash can be avoided without endangering third parties, traffic rules may be prioritized or disregarded, if necessary and depending on the individual situation.
- Correctly execute and actuate the driving plan: Driving plan should generate lateral and longitudinal control, based on driving plan and strategy.
- Communicate and interact with other road users: Automated driving vehicles are required to communicate and interact with other road users, depending on the specific ODD.
- Determine if specified nominal performance is not achieved: Factors such as unwanted human factors (misuse), deviation from the intended functionality, technological limitations, and environmental conditions influence nominal performance
- Ensure driver’s controllability: The driver’s (operator’s) level of control varies depending on the automation level. Whether or not there needs to be a driver depends on the level of automated driving and the ODD applying to the function.
- Detect when degradation is unavailable: Degradation unavailability must be identified, including the reason. Deactivation can only happen if driver/operator would be able to overtake, or vehicle could be put into a safe state.
- Ensure safe mode transitions and awareness: Ensure transitions are operated and controlled correctly. An example would be that the driver can only activate automated mode of car when inside the ODD, to be deactivated when leaving the ODD.
- React to insufficient nominal performance and other failures via degradation: Due to possibly unavailable nominal performance capabilities and other failures (e.g. hardware faults), the system should degrade within a well-defined amount of time (safe harbor).
- Reduce system performance in the presence of failure for the degraded mode: The reaction in case of failures during degraded mode should be defined.
- Perform degraded mode within reduced system constraints: Automated driving system operation in degrade mode occurs as nominal capabilities within limits. Multiple degraded capabilities should be possible, and a time frame for additional reaction is required.
Proper driving strategy
Considering all the above, a proper driving strategy needs to be developed. The driving strategy should be to drive in a collision-free manner without compromising comfort or traffic flow. Rules that need to be complied with are:
- Explicit traffic rules applying to the specific ODD in which the car maneuvers
- Implicit traffic rules,
i.e., the rules that are included in every set of traffic laws, or that apply
simply to automated driving because of its imminent danger and the intention of
the car manufacturer to avoid accidents, such as:
- Avoid collisions
- When in doubt, right of way is given, not taken (safety first!)
- Be cautious towards vulnerable road users (pedestrians, bicycle drivers, motorbike drivers)
- Prefer the safe maneuver to escape an unsafe situation
The automated vehicle should properly respond to dangerous situations, should not create collisions based on its own actions, and should be able to avoid collision even if confronted with unsafe behavior of other road users.
Sensors
An AV’s general capability to “sense and react” depends, above all, on its sensors.
Environment perception sensors are (i) the cameras, the lidar (light detection and ranging, high precision measurement of structured and unstructured elements), the radar (detection and ranging of moving objects), the ultrasonic (a nearfield sensor also known from the medical sector) and microphones (for acoustic signals such as a police siren or horns). The sensors need to be able to detect what they are supposed to detect under the given ODD—just as a human being would do in traffic.
Sensor fusion is the combining of sensory data or data derived from disparate sources such that the resulting information has less uncertainty. More than just one sensor system needs to provide for the identical information, with the highest probability of truth (bearing in mind that a 100 percent probability is impossible to reach and depending on whether action or omission is in question such probability thresholds may be different).
Generic information needs to be provided by HD mapping providing reliable information to the car on information that the car cannot see with its sensor, or to verify localization and positioning.
Global navigation satellite system (GNSS) refers to a constellation of satellites providing signals from space that transmit positioning and timing data to GNSS receivers. The receivers then use this data to determine location.
Vehicle-to-everything (V2X) communication is the passing of information from a vehicle to any entity that may affect the vehicle, and vice versa. It is a vehicular communication system that is not available everywhere yet. Every information needs to be accomplished by data on the motion of the vehicle itself (ego-motion) which describes the actual state of the car, including acceleration, deceleration, speed, etc.
Verification, validation…and post-deployment observation
The purpose of verification and validation (V&V) is verify all requirements derived through the safety-by-design strategy. One hundred percent reliability is impossible. Repeated testing is required. A third step, post-deployment observation (i.e. field monitoringand security of the automated driving system), is also recommended.
Key V&V challenges include:
- The statistical demonstration of system safety and a positive risk balance without driver interaction
- The system safety with driver interactions
- The consideration of scenarios currently not known in traffic
- The validation of various system configurations and variants
- The validations of (sub) systems that are based on machine learning
Simulation is key to effective V&V. Simulation helps to understand behavior of traffic participants (including the vehicle itself) and the outcome of a system in a virtual setting. Contrary to the real world, the system can be controlled, and scenarios can be created. Simulation serves to develop a function and to validate the function before it is released to the market. Simulation usage depends on the validation strategy, the requirements and the regulatory environment. Simulation relies on scenarios that derive from safety requirements, collected systematically through real-world driving, known crash scenarios, ODD infrastructure, and an informed brainstorm of the individuals involved, considering the systems weaknesses. Statistical confidence requires the simulation of many scenarios. The validity of the simulation needs to be tested for a subset of corner cases against real-world experience. It should be noted that gathering the real world data important for realistic traffic scenarios and accurate has raised substantial data privacy concerns, an issue that still needs to be resolved.
Five challenges, five solutions
Challenge No. 1: Statistical demonstration of system safety and a positive risk balance without driver interaction
- Utilize a statistical grey box testing during driving tests to provide for real-world driving scenarios to cultivate the following: statistical validation of the perception in real-world tests with final perception hardware in vehicles; validation of complete closed-loop system preferably in real-world driving conditions; and identification of driving scenarios
- Implement scenario-based testing for the full driving system as well as specific elements in test platforms using test techniques. These techniques include software/hardware reprocessing to validate perception and sensor fusion; validate trajectory-planning and control algorithms in simulations with basic sensor models covering a wide range of variations in the scenarios; conduct failure tests of hardware components, tests of fault injections, and validation of simulation; and Proving ground to validate critical traffic systems.
- Ensure field monitoring of the system over its lifetime to quantify and assess previously unconsidered scenarios and increase the confidence level of the validation with higher statistical power.
Challenge No. 2: System safety with driver interactions
- Because it is inevitable that a scenario would occur where human must take over the driving system, assessment of such scenarios must take place. Thus, the authors recommend the following steps as an example of such a sequence:
- Simulation to find worst-case traffic scenarios using a basic driver model;
- Driver in loop testing of driver performance in combination with human machine interaction;
- Real-world testing on the proving ground with a closed loop Level3/Level4 system with a safety driver;
- Real-world testing on the proving ground with a closed-loop Level3/Level4 system with expert drivers only (no safety driver);
- Real-world testing on the proving ground with a closed-loop Level3 or Level4 system with a representative sample of trained customers and an incremental increase of the ODD and drivers;
- Real-world testing described in (c) and (e);
- Reduced training of customers, and activation of the system in the full ODD;
- Field monitoring of system performance in the customer fleet (open-road testing, naturalistic driving studies).
- However, there is more assessment that could be done. Customer case studies can be conducted to demonstrate the level of vehicle controllability that the driver has for the known scenarios and to demonstrate that the defined response times are adequate, specifically for the Level3 vehicles.
Challenge No. 3: Consideration of scenarios currently not known in traffic
- To tackle this challenge, the human driver in relation to the entire ADS needs to be examined. Thus, testing platforms should rest on state-of-the-art strategies:
- Simulation with bidirectional interaction of a fleet of automated vehicles, and DIL and open-road testing to assess unknown scenarios.
- Driver in the loop and open-road testing to assess unknown scenarios resulting from the interaction of human drivers in Level 0 to Level 2 vehicles with automated vehicles.
- In both platforms, the behavior of the simulated automated vehicles needs to cover a broad range of possible system implementations. Although different manufacturers with different system characteristics might result in different scenarios, a plant model could be modified with different parameterizations to cover this aspect.
Challenge No. 4: Validation of various system configurations and variants
Regression testing combats complexity of the automated driving system because such testing would focus on changes between configurations. Moreover, full traceability along the complete development process identifies elements and software components affected by small changes, for e.g., in one line of code. For every change in one line of code, the elements and capabilities affected need to be identified. Consequently, testing can focus on the impact the change has on the affected capabilities compared to the previously tested baseline configuration.
Challenge No. 5: Validations of systems that are based on machine learning
Because it is important to verify and validate safety-related machine learning algorithms, it is vital to define a safe design process for algorithms in addition to testing them. Machine learning algorithms consist of deep neural networks, which include 3D object detection such as cameras and lidar-based technology to detect 3D objects, box positions, and dimensions.
A viable test strategy responds to the key challenges in the V&V of automated driving systems by carefully breaking down the overall validation objective into specific test goals for every object under test and by defining appropriate test platforms and test design techniques.
USEFUL LINKS
- Check out Dentons’ newsfeed aggregating the latest stories on self-driving vehicles.
- Learn more about Dentons and The Driverless Commute
- Follow Dentons on LinkedIn and Twitter